
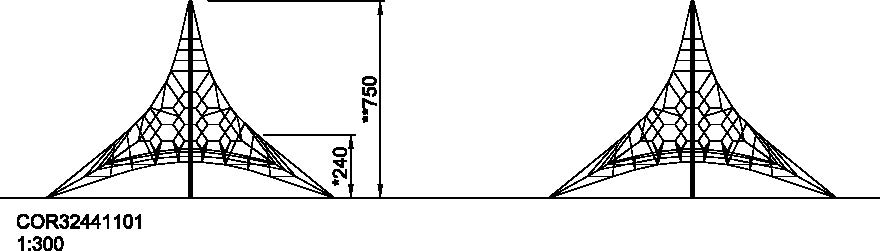
Training data for DetectNet consists of input images annotated with rectangular bounding boxes around objects to be detected. We followed the steps outlined in the DetectNet Parallel Forall post to train a modified version of DetectNet on the SpaceNet data. With the release of DIGITS 4 we also introduced an object detection network called DetectNet. There have been numerous deep learning approaches to object detection proposed recently two of the most popular are Faster RCNN and You Only Look Once (YOLO). Support for object detection was recently added in DIGITS 4. In an object detection approach we attempt to detect each individual building as a separate object and determine a bounding box around it. We will describe two approaches here and show some example results. There are multiple ways to use DIGITS to detect buildings in satellite images. The CSV provides the coordinates of the vertices of the building footprint polygons in latitude and longitude in the same map projection as the images, and the GeoJSON provides this same information in pixel coordinates relative to the image. The building footprints are provided in both CSV and GeoJSON formats. This enables precise mapping of each pixel in the image to a location on Earth. This is a public domain metadata standard which allows georeferencing information-such as map projections-to be embedded within a TIFF file. The image data is provided in GeoTIFF format. Figure 2: Example 3-band SpaceNet image and corresponding building footprints. Most buildings are quadrilateral but there are more complex building footprints throughout the dataset. The dataset shows a variety of different environments, with dense urban areas that have many buildings very close together and sparse rural areas containing buildings partially obstructed by surrounding foliage. There are also blank areas in some images that contain no pixel information at all (right-hand side of Figure 1). Some images contain more than 200 buildings, while others contain none. The dataset includes the polygons outlining all building footprints in each image, as Figure 2 shows. Figure 1: Example 3-band SpaceNet imagery. Figure 1 shows two example 3-band images. In this post we will focus solely on the 3-band images.

For more information on the spectral imaging capabilities of Worldview-2 see. This extended range of spectral bands allows Worldview-2 8-band imagery to be used to classify the material that is being imaged. The 8-band multispectral images contain spectral bands for coastal blue, blue, green, yellow, red, red edge, near infrared 1 (NIR1) and near infrared 2 (NIR2). 3-band Worldview-2 images are standard natural color images, which means they have three channels containing reflected light intensity in thin spectral bands around the red, green and blue light wavelengths (659, 546 and 478 nanometres (nm) respectively). Worldview-2 is sensitive to light in a wide range of wavelengths. Each image covers 200m 2 on the ground and has a pixel resolution of ~50cm.
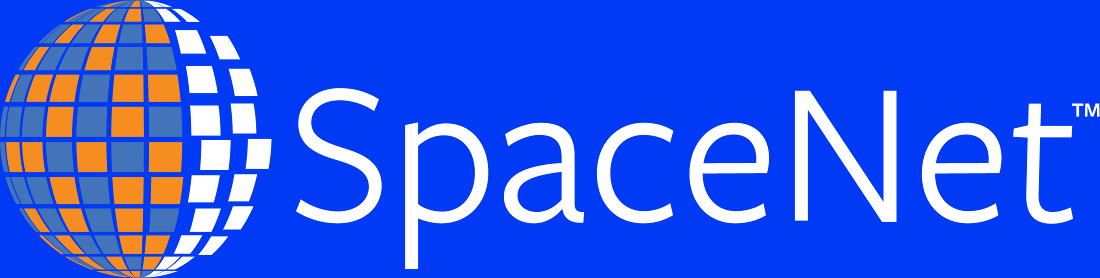
The first Area of Interest (AOI) released in the SpaceNet dataset contains two sets of over 7000 images by the DigitalGlobe Worldview-2 satellite over Rio de Janeiro, Brazil. We hope that this demonstration of automated building detection will inspire other novel applications of deep learning to the SpaceNet data. In this post we demonstrate how DIGITS can be used to train two different types of convolutional neural network for detecting buildings in the SpaceNet 3-band imagery. NVIDIA is proud to support SpaceNet by demonstrating an application of the SpaceNet data that is made possible using GPU-accelerated deep learning. State-of-the-art Artificial Intelligence tools like deep learning show promise for enabling automated extraction of this information with high accuracy. This information can be used in important applications like real-time mapping for humanitarian crisis response, infrastructure change detection for ensuring high accuracy in the maps used by self-driving cars or figuring out precisely where the world’s population lives. The SpaceNet release is unprecedented: it’s the first public dataset of multi-spectral satellite imagery at such high resolution (50 cm) with building annotations.
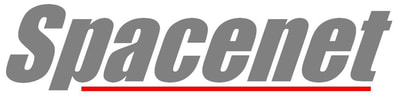
This public dataset of high-resolution satellite imagery contains a wealth of geospatial information relevant to many downstream use cases such as infrastructure mapping, land usage classification and human geography estimation. DigitalGlobe, CosmiQ Works and NVIDIA recently announced the launch of the SpaceNet online satellite imagery repository.
